
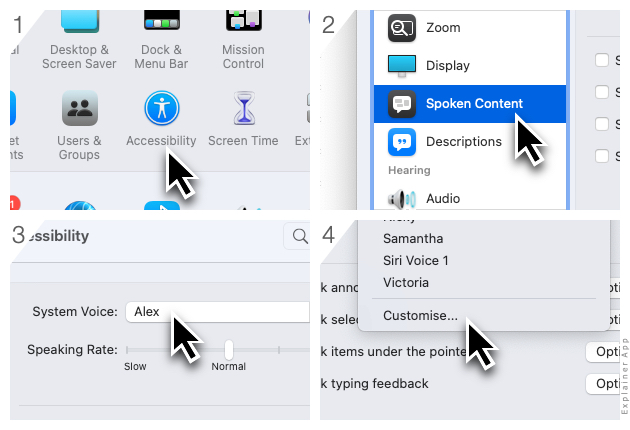
TTS model on LibriTTS datasets and fine-tune it on VCTK and LJSpeech datasets Part in addition to speaker embedding for adaptation. Normalization in the mel-spectrogram decoder of AdaSpeech, and fine-tune this 2) To better trade off theĪdaptation parameters and voice quality, we introduce conditional layer Predictor to predict the phoneme-level vectors. Phoneme-level vectors from the target speech during training in inference, weĮxtract the utterance-level vector from a reference speech and use an acoustic Two acoustic encoders to extract an utterance-level vector and a sequence of We design several techniques in AdaSpeech to address the twoĬhallenges in custom voice: 1) To handle different acoustic conditions, we use
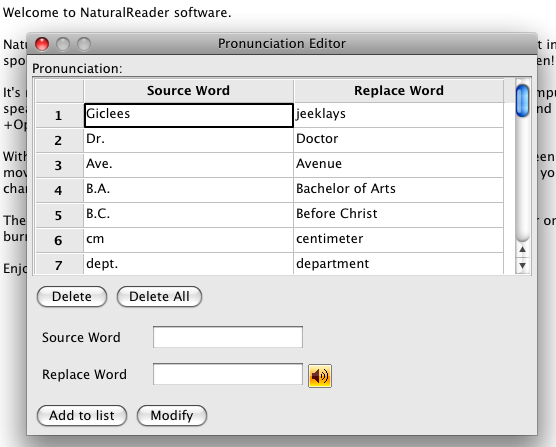
In this work, we proposeĪdaSpeech, an adaptive TTS system for high-quality and efficient customization Memory usage while maintaining high voice quality. Model needs to handle diverse acoustic conditions that could be very differentįrom source speech data, and 2) to support a large number of customers, theĪdaptation parameters need to be small enough for each target speaker to reduce Custom voice presents two uniqueĬhallenges for TTS adaptation: 1) to support diverse customers, the adaptation
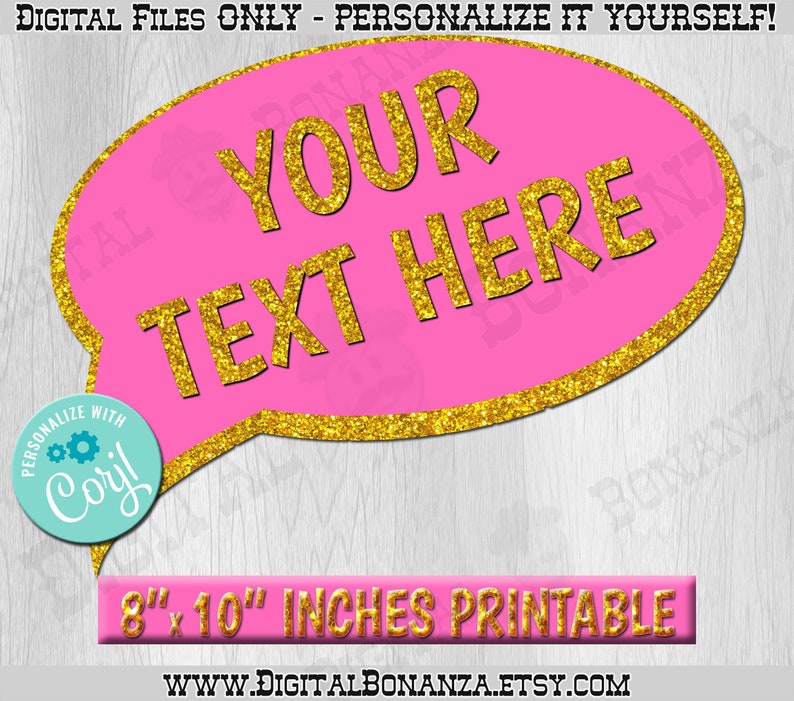
Platforms, aims to adapt a source TTS model to synthesize personal voice for a Download a PDF of the paper titled AdaSpeech: Adaptive Text to Speech for Custom Voice, by Mingjian Chen and 6 other authors Download PDF Abstract: Custom voice, a specific text to speech (TTS) service in commercial speech
